Table Of Content
Instead, it involves measuring several variables, often both categorical and quantitative, and then assessing the statistical relationships among them. These included health, knowledge of heart attack risk factors, and beliefs about their own risk of having a heart attack. They found that more optimistic participants were healthier (e.g., they exercised more and had lower blood pressure), knew about heart attack risk factors, and correctly believed their own risk to be lower than that of their peers. As with simple designs with only one independent variable, factorial designs have the same basic empirical question.
Replies to “A Complete Guide: The 2×2 Factorial Design”
There are situations where you have unequal variation for maybe a known reason or unknown reason, but if you have repeated observations and you can get weights, then you can do a weighted analysis. Is there always a transformation that can be applied to equalize variance? Although non-parametric methods have fewer assumptions about the distribution, you still have to worry about how you are measuring the center of the distribution. When you have a non-parametric situation you may have a different shaped distribution in different parts of the experiment.
5.2. Assessing Relationships Among Multiple Variables¶
The next step is selecting which terms will be analyzed for the responses. To do this, click on "Terms..." and the following menu will appear. The IV would be Mindset, with the levels being growth mindset or fixed mindset. As expected, we the average height is 6 inches taller when the subjects wear a hat vs. do not wear a hat. So, the main effect of wearing hats is to add 1 inch to a person’s height. Explore Psychology provides information about psychology and mental health.
The ever changing looks of Cheryl, including THAT X-factor design disaster and her fashion crimes with... - The Sun
The ever changing looks of Cheryl, including THAT X-factor design disaster and her fashion crimes with....
Posted: Wed, 21 Feb 2024 08:00:00 GMT [source]
Visualizing Main Effects & Interaction Effects
You need to have some understanding of what your factor is to make a good judgment about where the levels should be. In the end, you want to make sure that you choose levels in the region of that factor where you are actually interested and are somewhat aware of a functional relationship between the factor and the response. This is a matter of knowing something about the context for your experiment. When choosing the levels of your factors, we only have two options - low and high.
Use the Yates method in order to determine the effect each variable on the students performance in the course. Like Pareto plots, Half Normal plots show which factors have significant effects on the responses. The factors that have significant effects are shown in red and the ones without significant effects are shown in black. The further a factor is from the blue line, the more significant effect it has on the corresponding response. For wt% methanol in biodiesel, RPM is further from the blue line than pressure, which indicates that RPM has a more significant effect on wt% methanol in biodiesel than pressure does.
3.10. Interpreting main effects and interactions¶
This will allow you to determine the effects of temperature and pressure while saving money on performing unnecessary experiments. Suppose you have two variables \(A\) and \(B\) and each have two levels a1, a2 and b1, b2. You would measure combination effects of \(A\) and \(B\) (a1b1, a1b2, a2b1, a2b2).
Lesson 5: Introduction to Factorial Designs
The table above gives the data with the factors coded for each of the four combinations and below is a plot of the region of experimentation in two dimensions for this case. Suppose that you are looking to study the effects of hours slept (A), hours spent with significant other (B), and hours spent studying (C) on a students exam scores. You are given the following table that relates the combination of these factors and the students scores over the course of a semester.
1: Factorial Designs
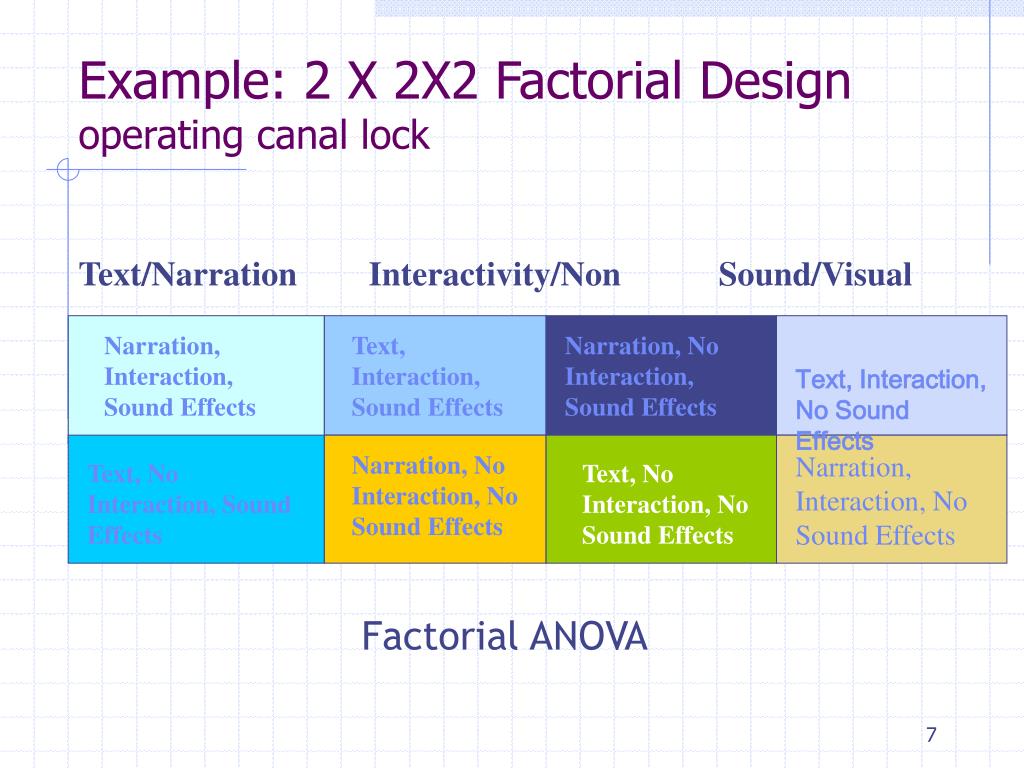
However, let’s imagine that she is also interested in learning if sleep deprivation impacts the driving abilities of men and women differently. She has just added a second independent variable of interest (sex of the driver) into her study, which now makes it a factorial design. We have first discussed factorial designs with replications, then factorial designs with one replication, now factorial designs with one observation per cell and no replications, which will lead us eventually to fractional factorial designs. This is where we are headed, a steady progression to designs with more and more factors, but fewer observations and less direct replication. In the Graphs menu shown above, the three effects plots for "Normal", "Half Normal", and "Pareto" were selected.
1. Multiple Dependent Variables¶
Each of the inferential statistical analyses that we've covered is to compare means (quantitative variable) between different groups. The qualitative variable is the two different samples or an IV with two or more levels. With t-tests, with compared two different groups (which can be considered one IV with two levels). Sometimes, those groups were unrelated (independent t-test) and sometimes they were related (dependent t-test). Sometimes those IV levels were unrelated (Between Groups ANOVA) and sometimes they were related (Repeated Measures ANOVA, sometimes called Within-Groups ANOVA). Certainly any research evaluation of intervention effectiveness can pose analytic and interpretive challenges.
As with any statistical experiment, the experimental runs in a factorial experiment should be randomized to reduce the impact that bias could have on the experimental results. Similar definitions hold for interactions of more than two factors. In the 2 × 3 example, for instance, the pattern of the A column follows the pattern of the levels of factor A, indicated by the first component of each cell. If the number of combinations in a full factorial design is too high to be logistically feasible, a fractional factorial design may be done, in which some of the possible combinations (usually at least half) are omitted. The normal probability plot of the effects shows us that two of the factors A and C are both significant and none of the two-way interactions are significant.
But the experimenters also know that many people like to have a cup of coffee (or two) in the morning to help them get going. For example, imagine that researchers want to test the effects of a memory-enhancing drug. Participants are given one of three different drug doses, and then asked to either complete a simple or complex memory task. The point of this example is that although the B factor is not significant as it relates to the response, percentage of product defects - however if you are looking for a recommended setting for B you should use the low level for B.
Privacy by design a key factor in data protection - ITWeb
Privacy by design a key factor in data protection.
Posted: Thu, 14 Mar 2024 07:00:00 GMT [source]
Plotting the means is a visualize way to inspect the effects that the independent variables have on the dependent variable. In other words, sunlight and watering frequency do not affect plant growth independently. Rather, there is an interaction effect between the two independent variables. But the two 2-way interactions effects combined are no longer significant, and individually, the interactions are not significant here either. So, the log transformation which improved the unequal variances pulled the higher responses down more than the lower values and therefore resulted in more of a parallel shape. Now we are in a position where we can drop the interactions and reduce this model to a main effects only model.
When we look at the normal probability plot below, created after removing 3-way and 4-way interactions, we can see that now BD and BC are significant. The surface plot shows us the same interaction effect in three dimensions in the twisted plane. In addition you can ask Minitab to provide you with 3-D graphical tools that will allow you to grab these boxes and twist them around so that you can look at these boxes in space from different perspectives. These procedures are all 'illustrated in the "Inspect" Flash movie at the beginning of this section. In visually checking the residuals we can see that we have nothing to complain about. There does not seem to be any great deviation in the normal probability plot of the residuals.
When you pick the correct transformation, you sometimes achieve constant variance and a simpler model. The tell-tale pattern that is useful here is an interaction that does not have crossing lines - a fanning effect - and it is exactly the same pattern that allows the Tukey model to fit. In both cases, it is a pattern of interaction that you can remove by transformation. If we select a transformation that will shrink the large values more than it does the small values and the overall result would be that we would see less of this fan effect in the residuals. Transformations towards the bottom of the list are stronger in how they shrink large values more than they shrink small values that are represented on the plot.
Finally, the researchers asked participants to rate their current level of disgust and other emotions. The primary results of this study were that participants in the messy room were in fact more disgusted and made harsher moral judgments than participants in the clean room—but only if they scored relatively high in private body consciousness. In addition, the use of a large number of factors allows for built-in evaluations of the robustness of the main effects of the ICs.
No comments:
Post a Comment